Hector Palacios
Research Scientist, Artificial Intelligence
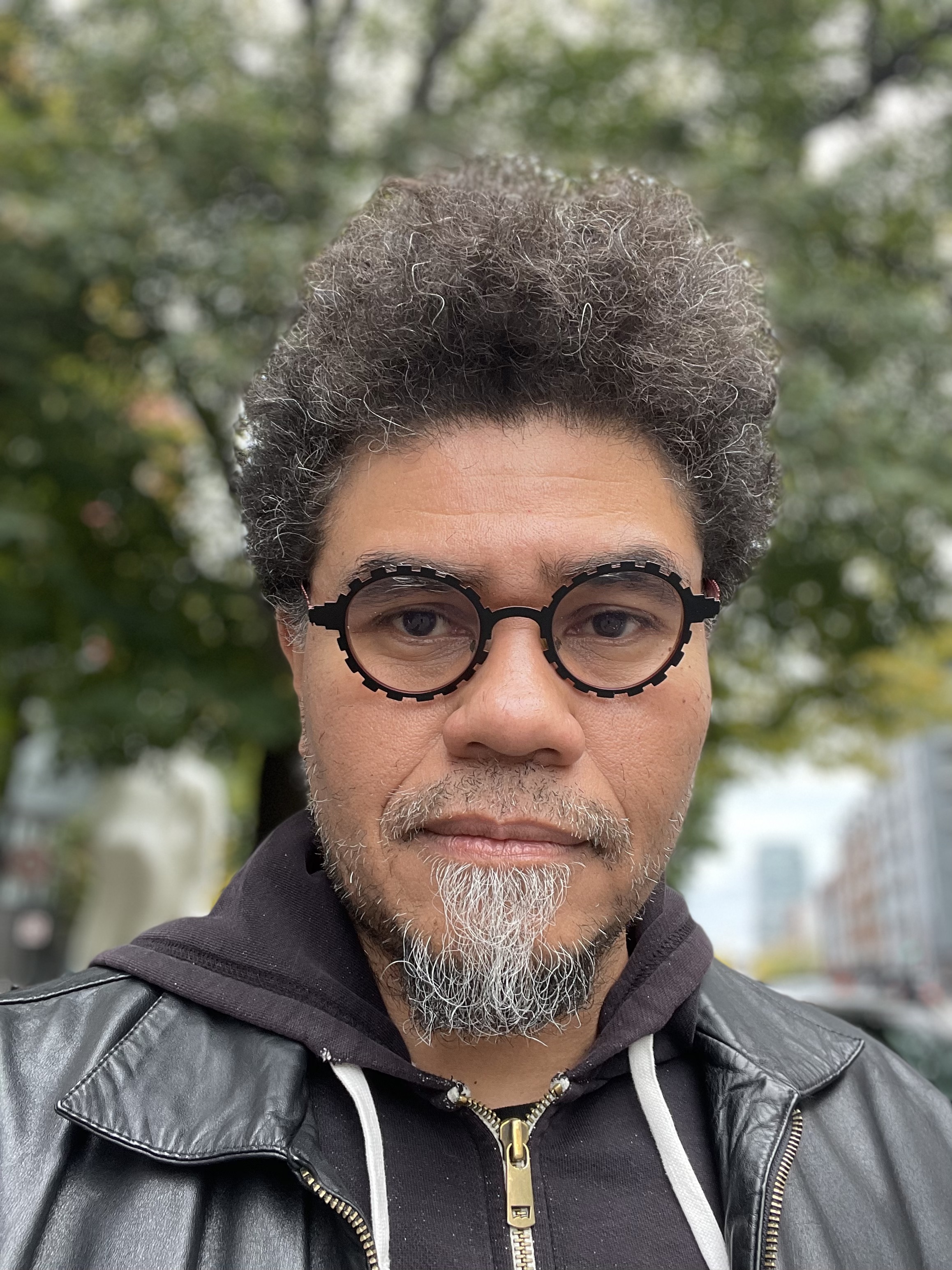
Montreal, Quebec, Canada
Hector is a research scientist specializing in NeuroSymbolic methods for Artificial Intelligence. His work specializes in problems where the output includes variable assignment like multi-labelled classification, and in sequential decision-making scenarios such as symbolic planning and task-oriented dialogue. Hector’s research blends machine learning and symbolic reasoning to address two main goals:
- First, to enhance the performance of pure machine learning methods by integrating symbolic reasoning, domain knowledge, context, and user-provided preferences.
- Second, to develop distributional methods with provable guarantees, striving for higher efficiency by tackling manageable subqueries or specific problem subclasses.
In pursuing these goals, Hector is interested in the principled combination of various algorithms and models, ranging from symbolic models to the outputs of pre-trained machine learning models like LLMs. Such combinations shed light on the nature of statistical approximations and the role of symbolic reasoning in AI.
In the realm of symbolic reasoning, Hector explores constraints, SAT, planning, and causal queries. Within machine learning, his interests lie in task-oriented dialogue and structured prediction. His applied research primarily centers on NLP, though he also has experience with vision models and multi-labelled classification models.
In recent years, Hector served as a research scientist at the ServiceNow Research Group, following the acquisition of ElementAI. Upon returning to academia, Hector garnered accolades for his work on AI reasoning, introduced innovative algorithms, and honed his expertise in melding AI techniques.
news
Apr 9, 2024 | Attending NeurIPS 2023 at New Orleans.accepted at NeurIPS 2023 https://jmlr.org/tmlr/editorial-board.html I’ll join Xiaotian Liu (University of Toronto) presenting our poster for the work _“Egocentric Planning for Scalable Embodied Task Achievement”, joint work with Christian Muise (Queen’s University). Join us at the poster session on December 12th, 2023 at New Orleans, 10:45 a.m. — 12:45 p.m. CST. More details at the NeurIPS 2023 site (login required)..Paper: https://openreview.net/forum?id=v0lkbp66Uw |
---|---|
Dec 4, 2023 | Attending NeurIPS 2023 at New Orleans.accepted at NeurIPS 2023 I’ll join Xiaotian Liu (University of Toronto) presenting our poster for the work _“Egocentric Planning for Scalable Embodied Task Achievement”, joint work with Christian Muise (Queen’s University). Join us at the poster session on December 12th, 2023 at New Orleans, 10:45 a.m. — 12:45 p.m. CST. More details at the NeurIPS 2023 site (login required)..Paper: https://openreview.net/forum?id=v0lkbp66Uw |
Sep 21, 2023 | “Egocentric Planning for Scalable Embodied Task Achievement” accepted at NeurIPS 2023Joint work with Xiaotian Liu (University of Toronto) and Christian Muise (Queen’s University).Abstract: Embodied agents face significant challenges when tasked with performing actions in diverse environments, particularly in generalizing across object types and executing suitable actions to accomplish tasks. Furthermore, agents should exhibit robustness, minimizing the execution of illegal actions. In this work, we present Egocentric Planning, an innovative approach that combines symbolic planning and Object-oriented POMDPs to solve tasks in complex environments, harnessing existing models for visual perception and natural language processing. We evaluated our approach in ALFRED, a simulated environment designed for domestic tasks, and demonstrated its high scalability, achieving an impressive 36.07\% unseen success rate in the ALFRED benchmark and winning the ALFRED challenge at CVPR Embodied AI workshop. Our method requires reliable perception and the specification or learning of a symbolic description of the preconditions and effects of the agent’s actions, as well as what object types reveal information about others. It is capable of naturally scaling to solve new tasks beyond ALFRED, as long as they can be solved using the available skills. This work offers a solid baseline for studying end-to-end and hybrid methods that aim to generalize to new tasks, including recent approaches relying on LLMs, but often struggle to scale to long sequences of actions or produce robust plans for novel tasks. Preprint: https://arxiv.org/abs/2306.01295 |
selected publications
- Egocentric Planning for Scalable Embodied Task AchievementNeurIPS 2023, poster., 2023Code+models at https://drive.google.com/file/d/1fI1Fq8ODLH3MGFVg4DMC0_XjoK4wBk-n/view?pli=1
- Improving Generalization in Task-oriented Dialogues with Workflows and Action PlansarXiv preprint arXiv:2306.01729, 2023
- Scaling up ML-based Black-box Planning with Partial STRIPS ModelsarXiv preprint arXiv:2207.04479, 2022
- A Knowledge Compilation Perspective on Queries and Transformations for Belief TrackingAnnals of Mathematics and Artificial Intelligence, 2023Accepted. Camera ready sent.